DINO v2: Match and transfer features across domains and understand relations between similar parts of different objects
"DINOv2: Learning Robust Visual Features without Supervision" - Research Paper Explained
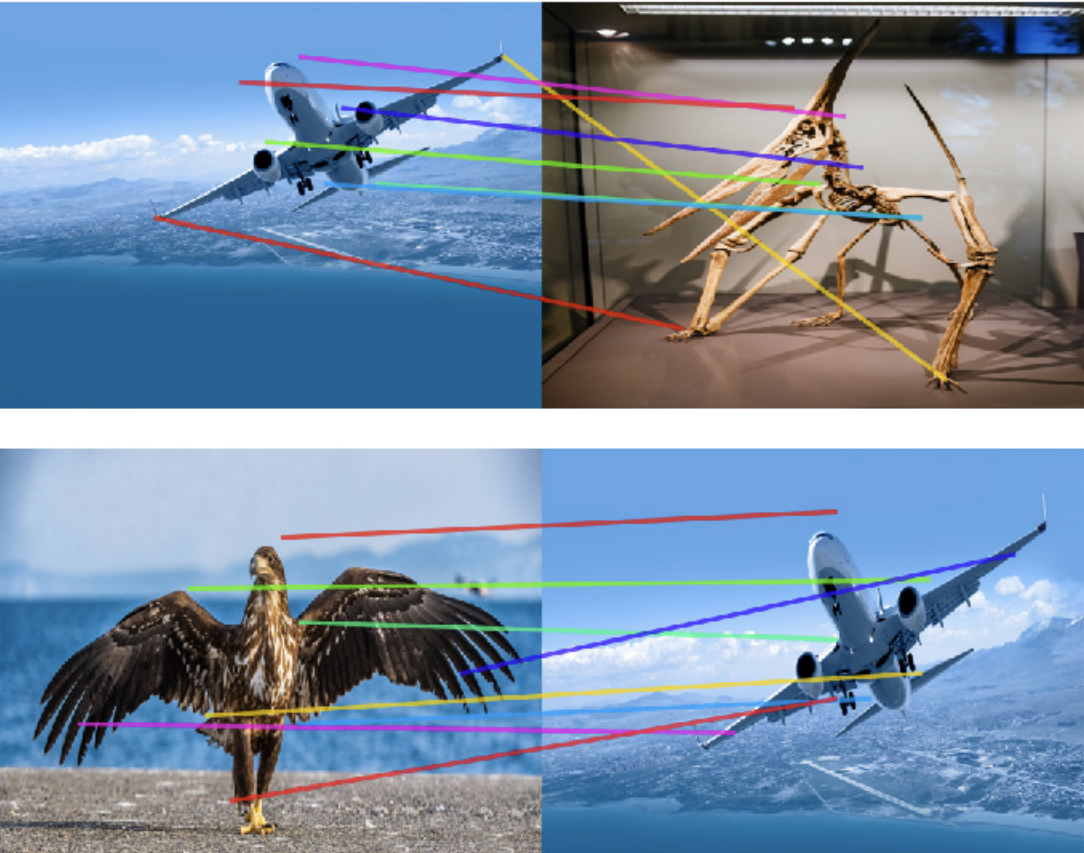
MetaAI DINOv2 is a foundation model for computer vision. DINOv2 paper shows that existing pretraining methods, especially self-supervised methods, can produce all purpose visual features, (i.e., features that work across image distributions and tasks without finetuning) if trained on enough curated data from diverse sources. It turns out that the approach of DINOv2 can match patch-level features between images from different domains, poses and even objects that share similar semantic information. This exhibits the ability of DINO v2 model to transfer across domains and understand relations between similar parts of different objects. The Meta shows its strengths and wants to combine DINOv2 with large language models.
more ...